The UK India Business Council, NASSCOM, and TechUK have published a Joint Position Paper on Enabling Data Transfers for India-UK Digital Trade. In the ongoing India-UK Free Trade Agreement negotiations, the paper provides a range of proposals for both governments to consider. Further, the paper will provide information to governments on their efforts to change domestic data protection regimes and cross-border data transfers and stimulate bilateral conversations aimed at reaching a data adequacy agreement. However, this is merely one of the cases today.
Many countries and states are passing, or have already passed, data privacy legislation, such as the PDPB by India, GDPR by the European Union, and the CCPA from the state of California. However, consumers have a right to know how their personal data is gathered, how it is utilised, and how long it remains stored in the database, especially with massive volumes of personal data being accumulated across many devices daily.
Artificial Intelligence, today, is being used for customer self-service bots, autonomous vehicles, employee HR portals, chatbots and virtual assistants, and many other applications. The question is how artificial intelligence (AI) can be leveraged to preserve customer privacy.
AI to aid privacy
Machine learning models are used to predict or infer sensitive personal information from the non-sensitive data of customers. Take, for instance; keyboard typing patterns can be used to deduce a person’s emotional state, likes and dislikes, as well as their political opinions, sexual orientation, and other such characteristics. AI systems can also utilise the available data as input to sort, score, classify, evaluate, or rate people, making it easier to discriminate against them for things like credit, housing, and employment. Fortunately, AI has the ability to improve privacy protection as well.
Automated data classification: Data classification, to be precise, is the process of separating and arranging data into appropriate groupings (“classes”) based on their shared features, such as their level of sensitivity and the dangers they pose, as well as the compliance requirements that protect them. Data can be classified into the following categories:
- Public data
- Confidential data
- Sensitive data
- Personal data
Now, both government organisations, companies and companies can effectively use data classification AI tools to accomplish data classification at scale. While a primary need is to create categories and outline a data classification procedure, data tagging can be wholly automated with machine learning. ML models are built to analyse and classify data by feeding them tagged datasets or training data. These systems will always apply the same criteria (and improve as they classify fresh data), making them more accurate at classifying data than humans.
Federated Learning: Federated learning, simply put, is the decentralised form of machine learning. Machine Learning models are trained on data collected from a variety of edge devices, such as mobile phones and laptops, and then eventually saved on a centralised server. Machine Learning systems then use this data and train themselves, eventually predicting outcomes for new data. But isn’t it a nightmare for privacy? It is well known that the AI market is dominated by big tech players such as Apple, Google, Microsoft, and Amazon, and they control most of the cloud-based solutions and APIs.
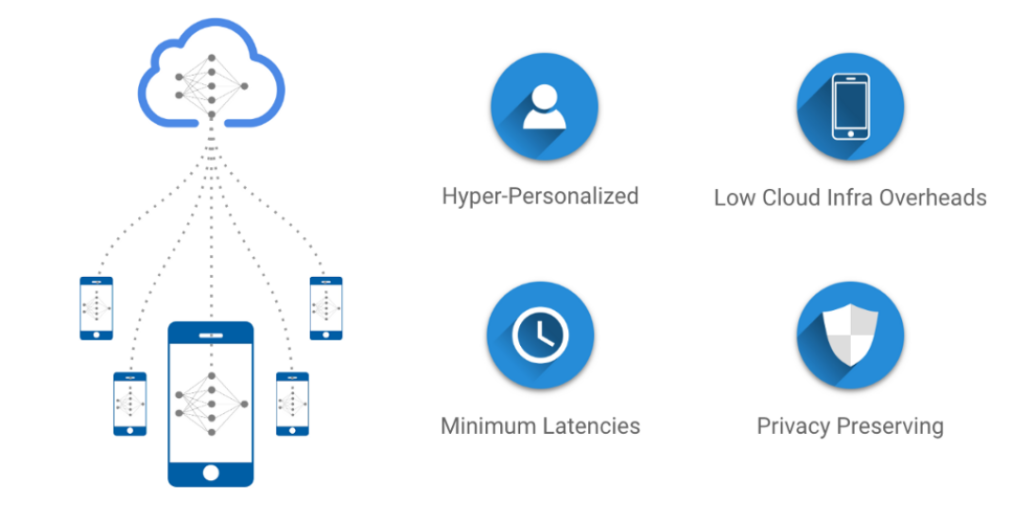
Source: Github
This is where federated learning comes in handy. Instead of a centralised server, the ML models will now train and learn on local devices. Over time as these models get smarter, only the results and not the data will get transferred to the centralised servers. This will build a system in which user-sensitive data is encrypted with an encryption key that is not held by any centralised cloud server.
Behavioural Analytics: Traditional technologies are less effective at detecting and responding to data breaches than AI. With its automated features, AI technology uses a more efficient approach and protects data by detecting malware through behaviour modelling and automated countermeasures. Behavioural analytics tools will construct a behavioural profile for each user based on the data collected over time. One can track and forecast patterns based on usage over time by merging this data with machine learning tools.
Conclusion
Over time, the awareness and concern around the loss or misuse of personal and private data, whether by companies on purpose or as a result of data breaches, are bound to see a significant rise. Although, with the rise of technology adoption and the capability of AI to erroneously expose consumer data, there are many opportunities for market players to reduce data breaches, standardise privacy practices and become more compliant with national and international data privacy regulations.
Source: indiaai.gov.in