Computer Vision models work across various modalities such as images, videos and depth perceptions. These models excel humans in the tasks that they are designed for. But one of the shortcomings that they possess is their lack of flexibility. To address this issue and make models more flexible, the Meta AI research team came up with a Computer Vision model called OMNIVORE. As the name suggests it is a single vision model that can operate across modalities. The performance of OMNIVORE is said to be better than conventional modality-specific models of the same size. This model developed by Meta AI has two major benefits
- It can perform cross-model generalisations. The model applies what it has learned from one modality to another modality.
- It is cost-effective and saves time on the research when compared to the ones functioning on modality-specific models.
OMNIVORE can be trained easily. While using ready-made standard datasets, the functioning of the model was equal to or higher than the corresponding single model.
Functioning of an OMNIVORE
The OMNIVORE is based on transformer architecture. Although the model is compatible with any transformer model, the base was built in Swin Transformer given its standout performance in image and video analytics. The functioning of an OMNIVORE can be understood through various stages.
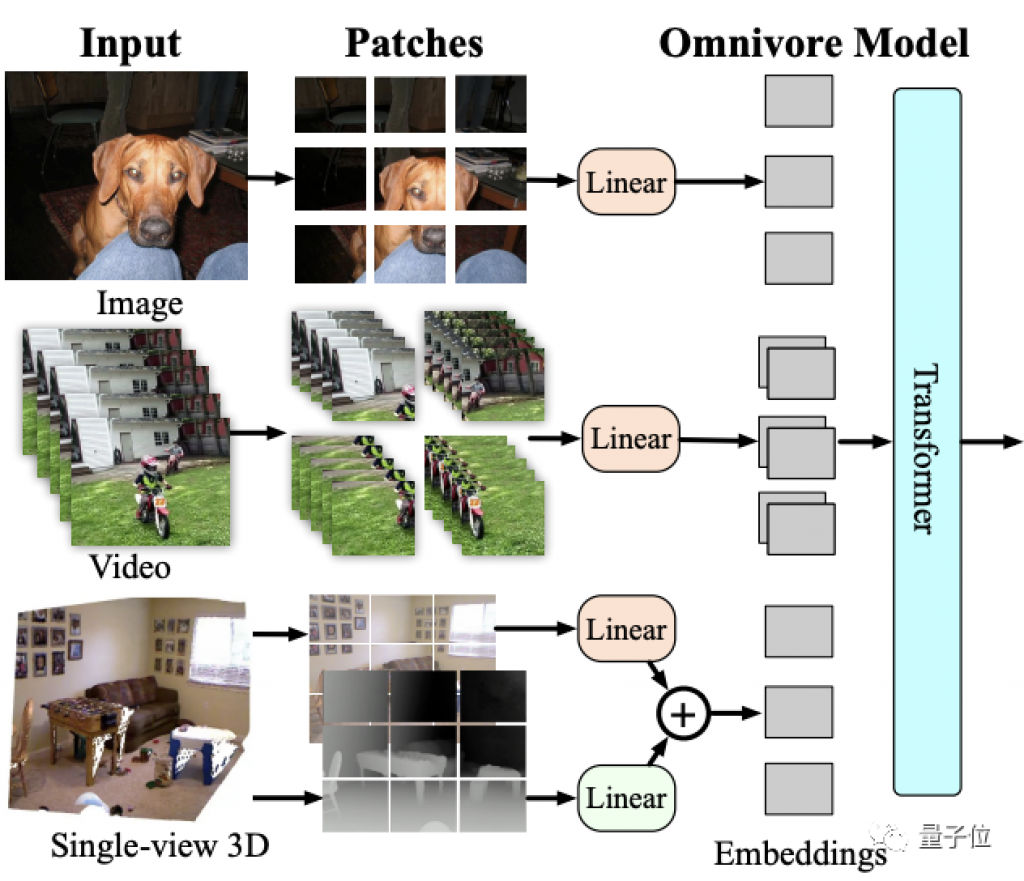
- Images, videos and single-view 3D modalities are converted into embeddings
- Embeddings are fed into the transformer model
- The transformer converts images into patches, video into patio-temporal labels and 3D images into RGB patches and depth patches
- With the use of a linear layer, the patcher is projected into embeddings
- The same linear layer is used for RGB patcher whereas a operate one is used for depth patches
The model converts all visual modalities into a common format through embedding. Later it uses a series of spatiotemporal attention operations to build a unified representation of varied modalities. According to the research team, they were surprised that even though the model did not undergo explicit training in cross model correspondence, OMNIVORE representation generalise well across all visual modalities. These capabilities of the model evolve without cross-modal supervision, due to parameter sharing between the models.
Experimenting OMNIVORE
Series of experiments were conducted on OMNIVORE. The researchers experimented comparing it with the modality-specific model. They considered three different model sizes: models T, S and B. The pre-trained model was fine-tuned on all seven tasks, whereas image specific models were pre-trained on 1N1K. The video-specific model and single view 3D-specific model were formatted using inflation of the other two models and they were fine-tuned on K400 and SUN RGB-D respectively. According to the Meta AI research, results stated that OMNIVORE can achieve 86.0% purity on ImageNet, 84.1% in the Kinetics dataset for action recognition and 67.1% on SUN RGB-D for single-view 3D scene classification. Also, the results confirmed that the performance of the model was better than or equal when in comparison. Among all the models, Swin-B was the model that achieved SOTA on all the tasks.
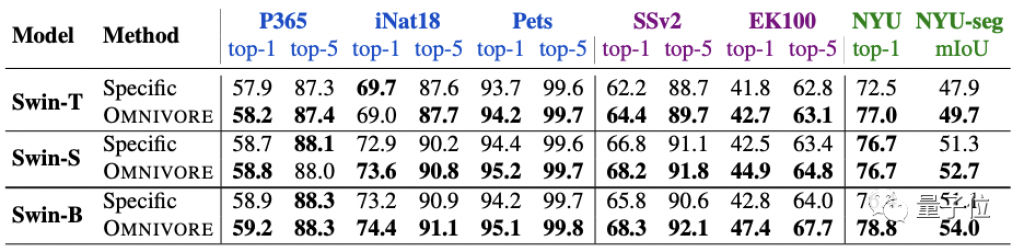
When the OMNIVORE was compared to a specific model which had the same architecture and number of parameters, the results were the same. Then the OMNIVORE was trained from scratch on 1N1K, K400 and SUN datasets and the VideoSwin and DepthSwin were fine-tuned from the ImageSwin model. The researchers next experimented the model with SOTA models on image, video and 3D data classification tasks. All the results were still good with OMNIVORE outstanding other models and showcasing better performance.
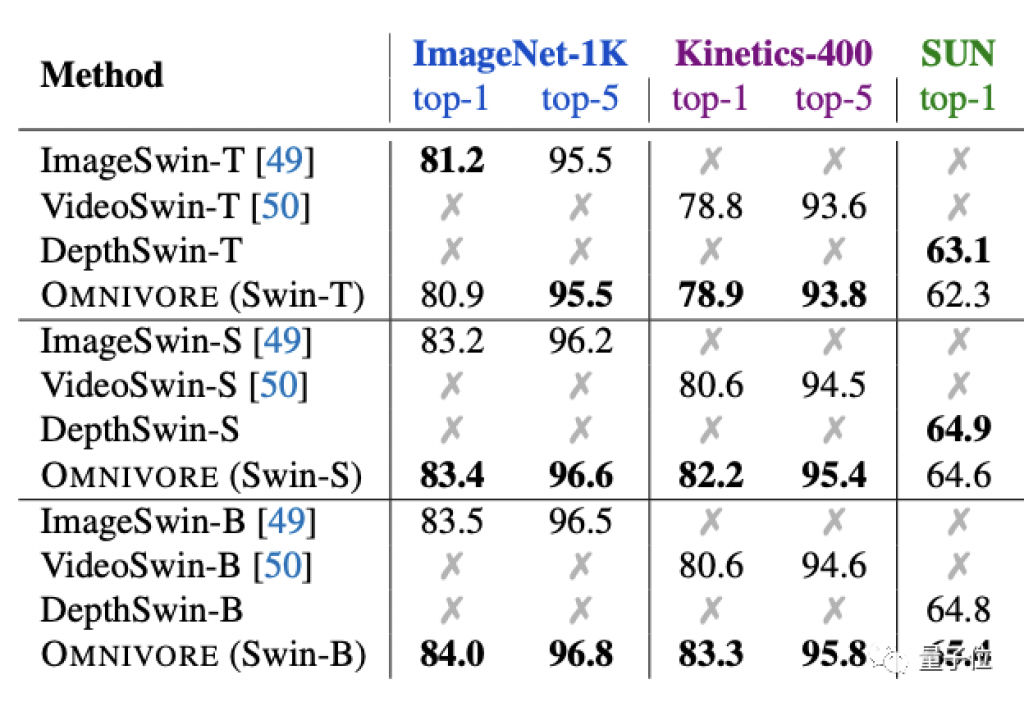
It was also found that even though the model was not trained on 1K depth maps, OMNIVORE was capable of providing semantically similar correct answers by retrieving depth maps. The researchers are confident that the model can overcome and tackle several limitations in the field of AI and computer vision.
Source: indiaai.gov.in